Predictive maintenance takes machine service to a new level. Using weMonitor, machine manufacturers and machine operators can keep an eye on all relevant machine data. Machine and plant monitoring are based on the latest internet technologies and intelligent data processing. weMonitor is thus the perfect basis for monitoring of machines and systems. In order to meet the individual requirements of the user, the software is individually configured and adapted. This ensures optimum machine and plant monitoring at all times.
In order to represent the entire system, weMonitor has future-oriented predictive maintenance functions. To implement predictive maintenance cost-efficiently, it is necessary to identify possible problems and malfunctions at an early stage. Therefore, machine learning algorithms are integrated which describe and analyze machines and plants with complex threshold values as a coherent system. In this way, machine learning enables knowledge to be generated independently, based on the experience gained. The predictive maintenance and repair reaches a new performance level, since the analysis of very large amounts of data is automated and solutions for new and previously unknown problems can be identified. In addition to machine learning methods that learn the vibration behavior of systems or components, we have also developed multidimensional anomaly detection methods whose learned patterns be executed on IoT Gateways. A wide range of options for recording and analyzing sensor data allows the weMonitor user to examine the system behavior to be monitored more closely during the development/commissioning phase. Predictive maintenance in conjunction with machine learning thus not only serves to increase plant availability, but also effectively reduces maintenance costs by predicting machine downtimes and defects.
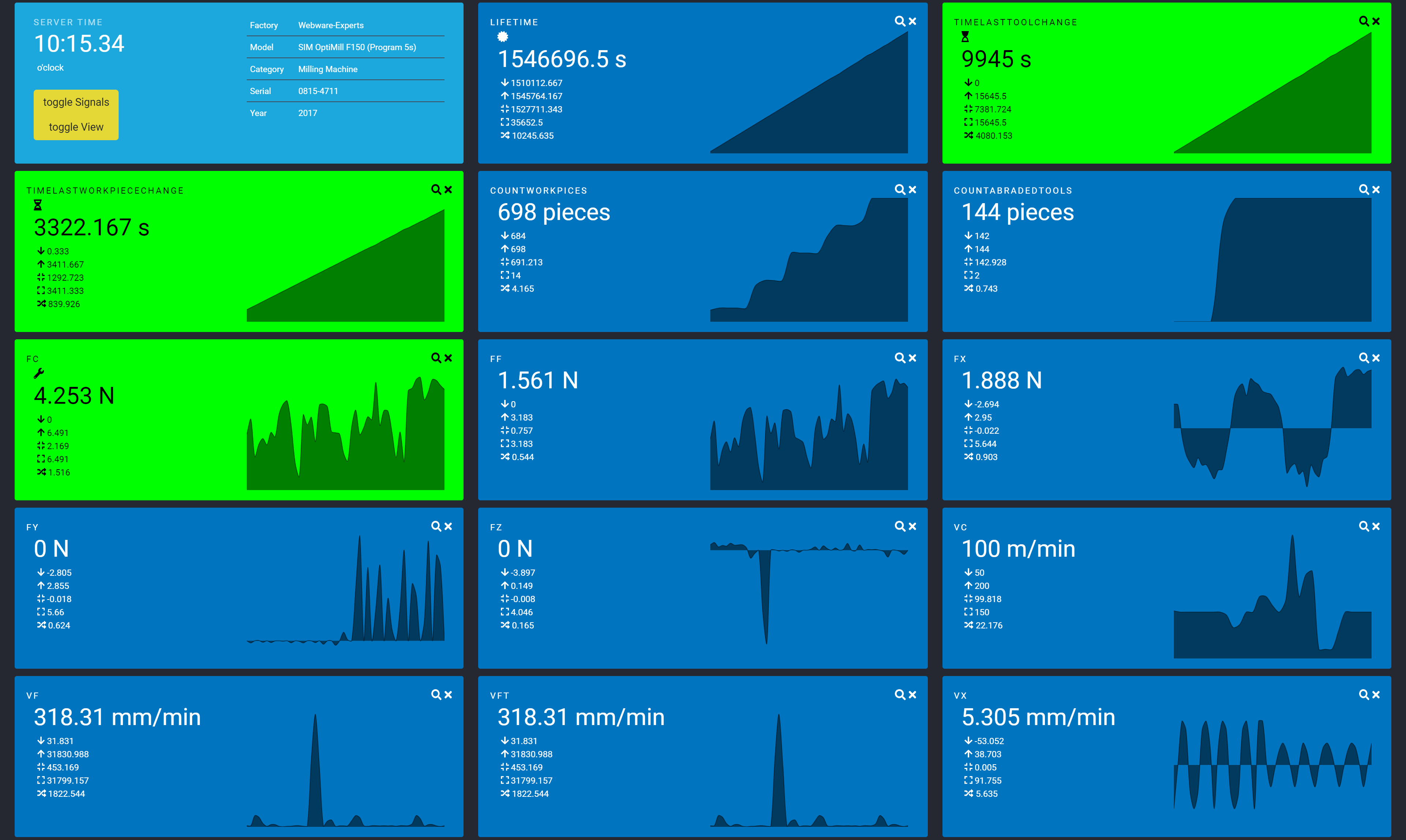
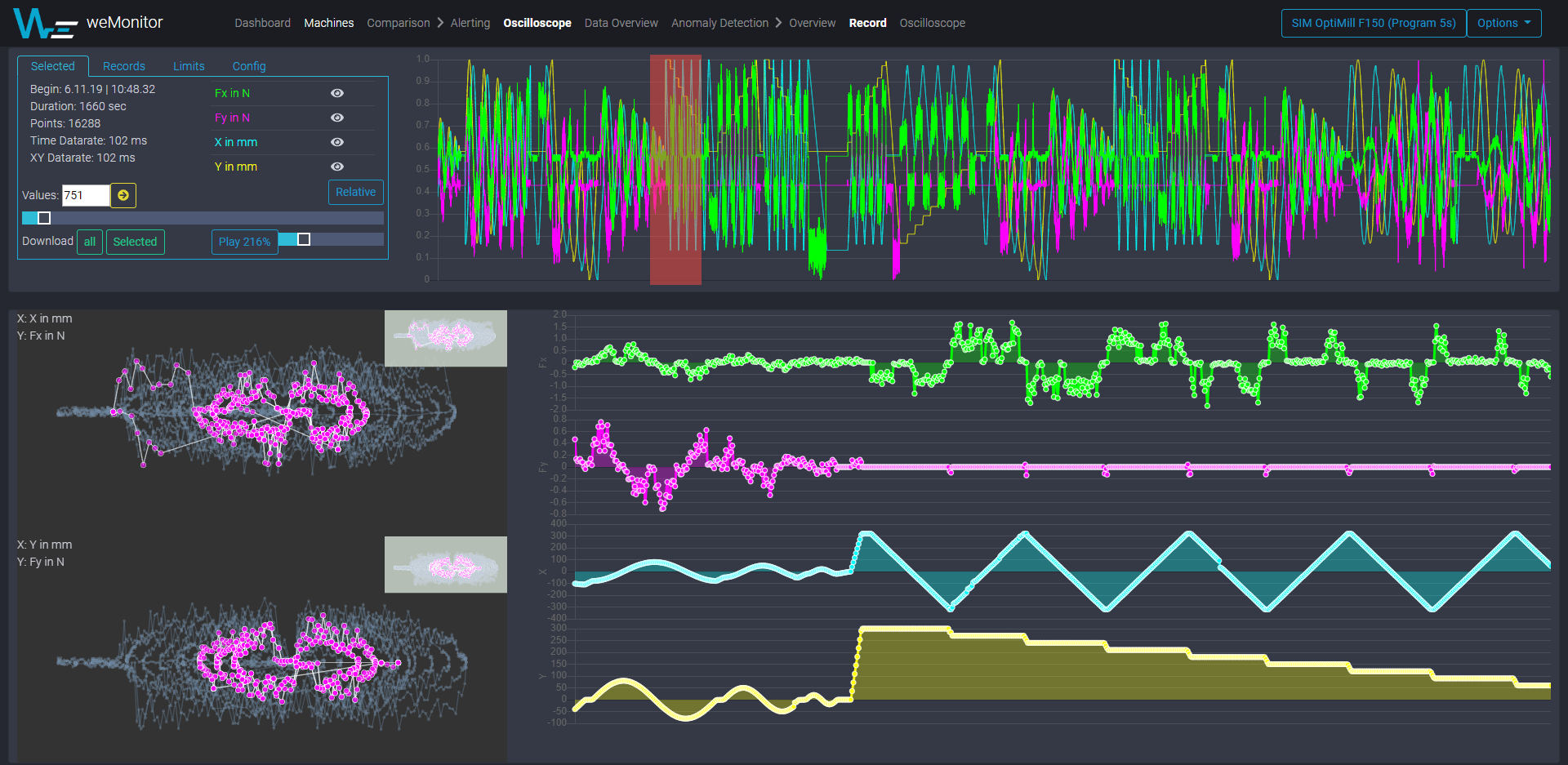
An essential component of condition monitoring is the display of data and sensor values in real time. Possible deviations are detected while further data is filtered out for more detailed analysis. The basis for condition monitoring is therefore precisely defined and individual threshold values. A prerequisite for successful data acquisition are machines and systems equipped with sensors in order to evaluate parameters such as vibrations, temperatures or other process and performance parameters both online and offline. The operator can individually adapt the module to his needs and define precise limit values, which trigger automatic alerts in the event of exceeding or falling short and generate alarm messages that are clearly visualized in the service platform. weMonitors condition monitoring is therefore ideally suited for optimum monitoring.
weMonitor not only increases the net operating time of the machines, but also increases profit and satisfaction among machine operators and users.
Application scenarios
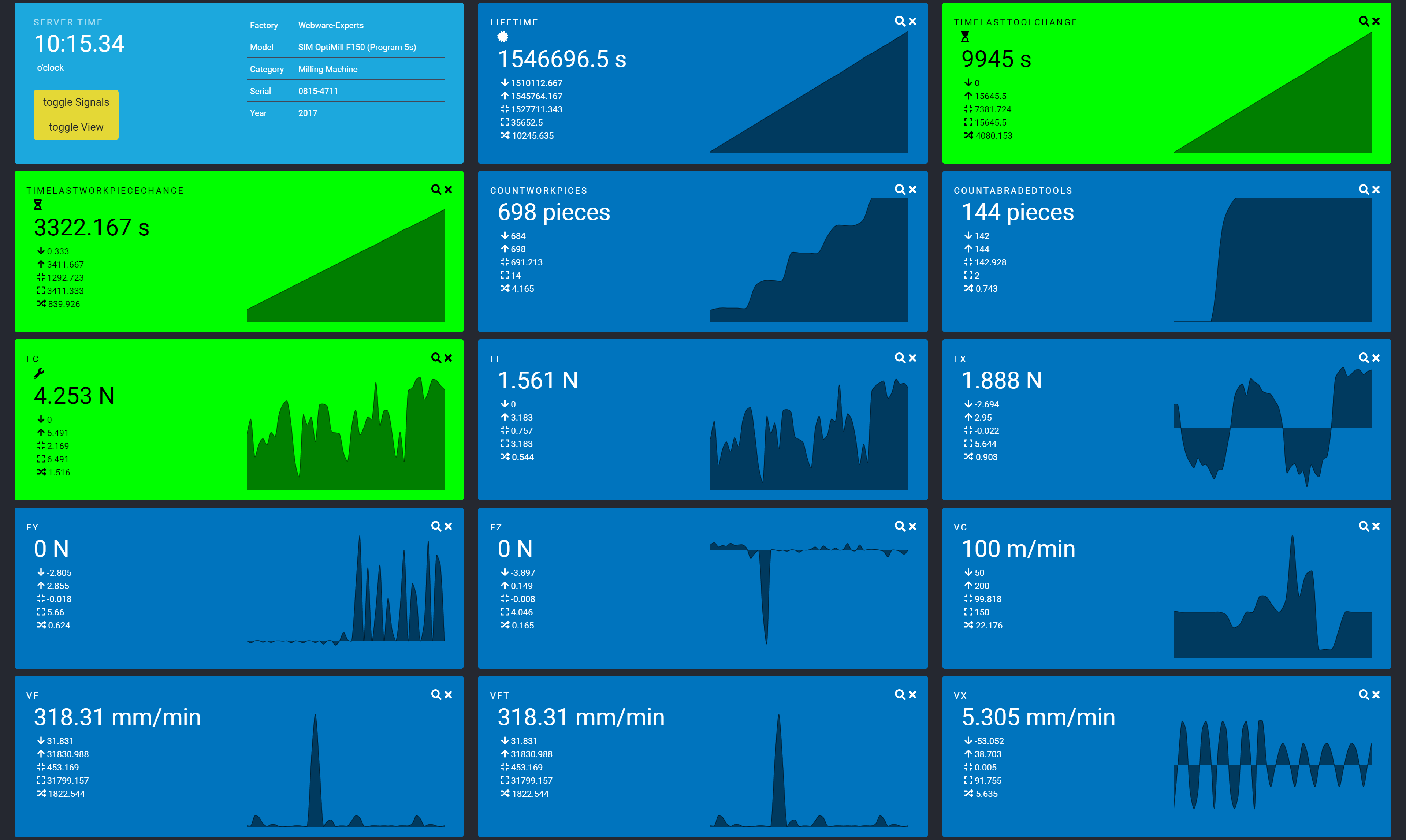
The real-time analytics of weMonitor offers different possibilities to check the condition of a machine. The menu item data overview with different view options gives a first impression. On the one hand the current numerical values of the machine are displayed. These are extended with minimum/maximum values average values, the minimum/maximum difference and the standard deviation. On the other hand, in the extended view the value progressions can be displayed with further details. To perform a comprehensive real-time analysis, weMonitor provides the oscilloscope, which is used for troubleshooting and subsequent signal analysis.
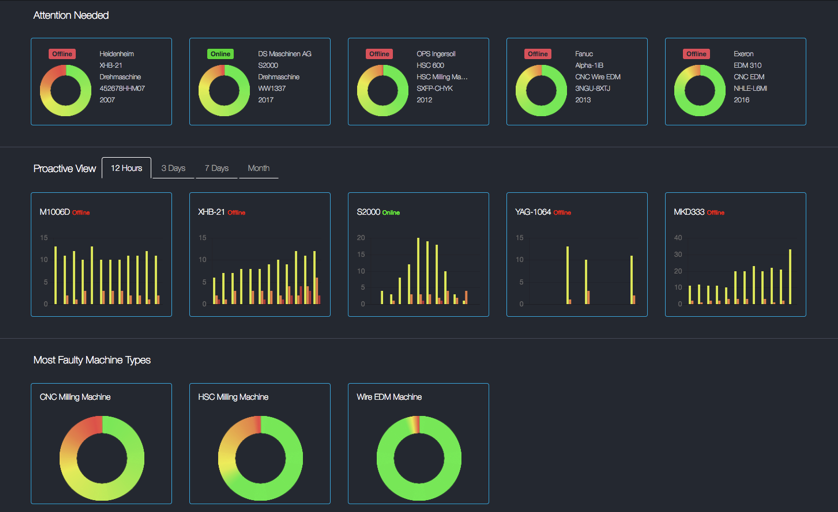
weMonitor provides users with an overview that enables them to carry out an efficient lifecycle management. The starting point is the central dashboard. It provides information that gives hints on how error-prone different machine types are. Using the permanently stored alerts and the associated sensor data, manufacturers are able to find the exact causes of more frequent failures or problems. Based on this information, improvement measures can be taken for the affected machine systems. A further plus is the better planning of maintenance for machine users. They can see critical machine types at a glance, which allows them to initiate countermeasures in good time.
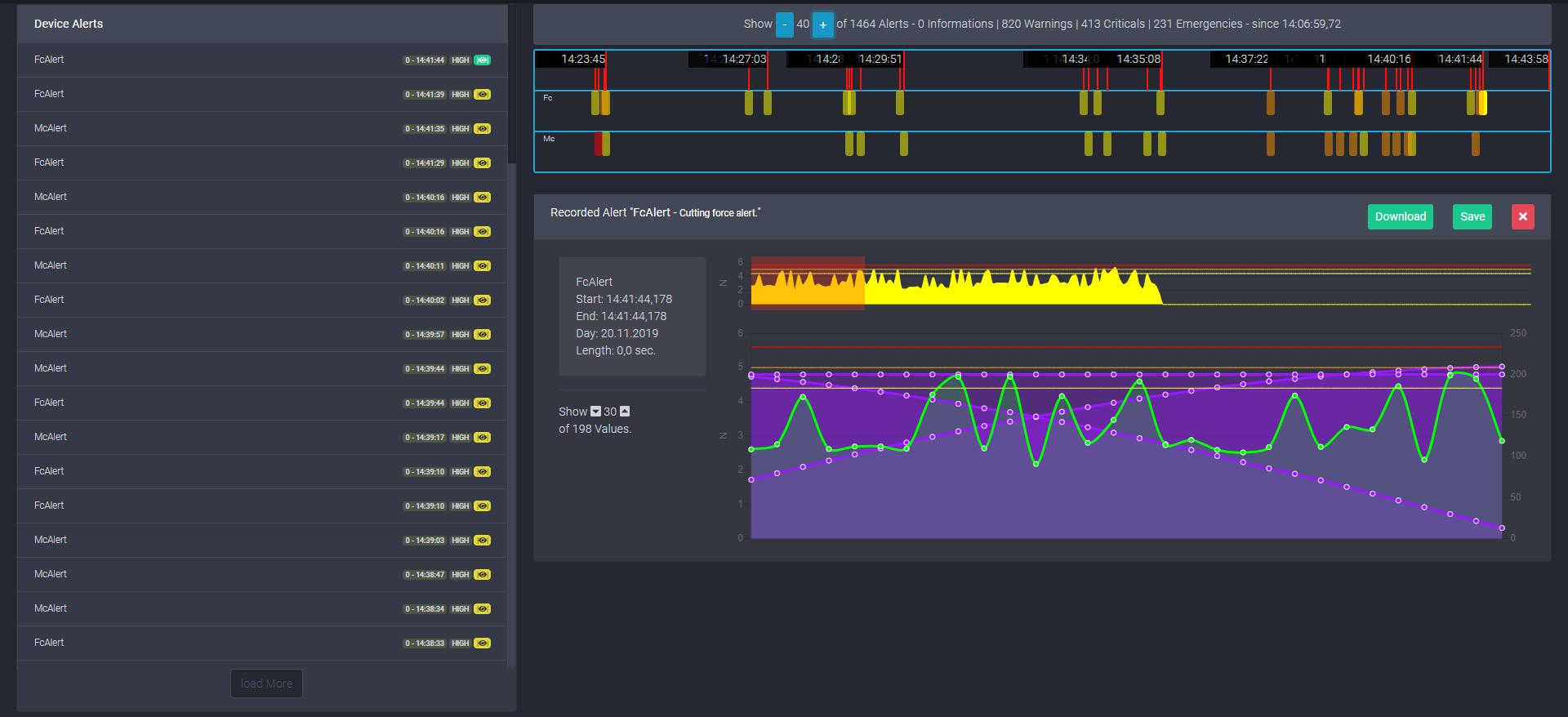
The module actively supports users in quality assurance. On the one hand, this can be done by reporting alerts at an early stage. For this purpose, the limit values of the sensors are defined in advance so that alerts with the severity Warning are issued if they are exceeded or undercut. On the other hand, predictive maintenance functionalities serve quality assurance. The aim is not only to carry out predictive maintenance using anomaly detection, but also to guarantee a consistently high quality of production as a result. Precise and timely knowledge of wear situations or of problematic developments in sensor values makes it possible to implement preventive maintenance before there is a loss of quality during operation.
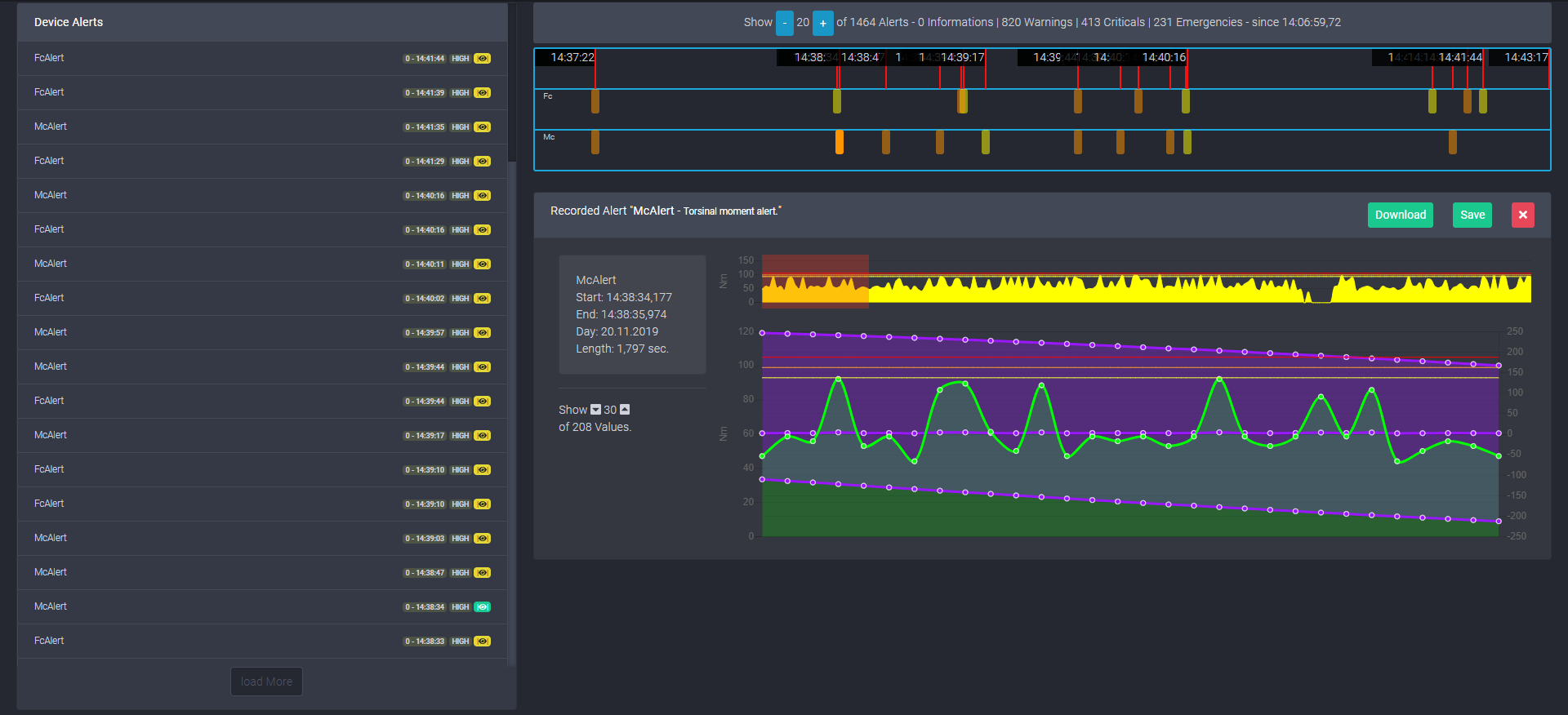
For the respective sensors of a machine it is possible to define alarm thresholds that should not be exceeded or fallen below. These can have the severity levels Warning, Critical and Emergency. In addition, related signals can be selected: they are directly related to the alert and are recorded and displayed accordingly in the event of an alarm. If an alert is generated, it appears in an overview list as well as in a time course diagram in the alerting menu option. When you select an alert in one of the two display formats, further detailed information about it is displayed.
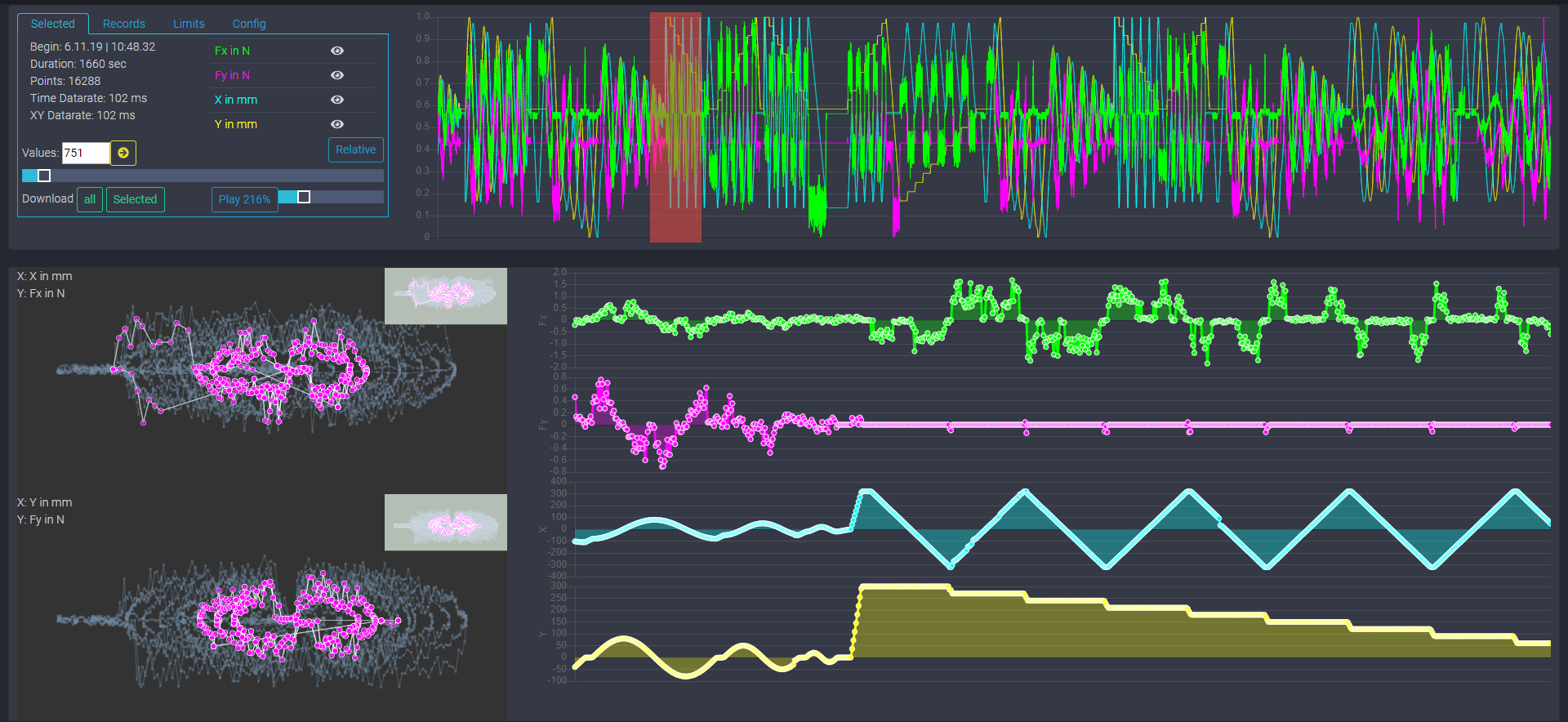
The different views of weMonitor are designed for different tasks. During troubleshooting several of these views can be combined. For example, sensor values saved as a record can be compared with current values. With this approach any deviations from optimal operation can be detected. Via the menu item Comparison, up to four different sensors can be compared. In the oscilloscope, the individual sensor values can be examined and analysed in detail. In this way they can be checked for plausibility and the sources of error can be located exactly. This more detailed signal analysis includes further sub-items in the application.
Signal analysis is the general functionality of weMonitor. A previously recorded record is analyzed here in the oscilloscope more precisely and used for troubleshooting. From the display of the complete value history it is possible to select self defined sections and have them displayed in the detail view. The size or the number of the listed values can be dynamically adjusted. In addition to selecting a desired passage, it is also possible to play back the record. The recorded data can also be exported as a CSV file and can be used in an external program for further analyses.
The signal analysis is further extended by three sub-items: weMonitor supports the user during development and commissioning as well as during the operation of machines and plants.
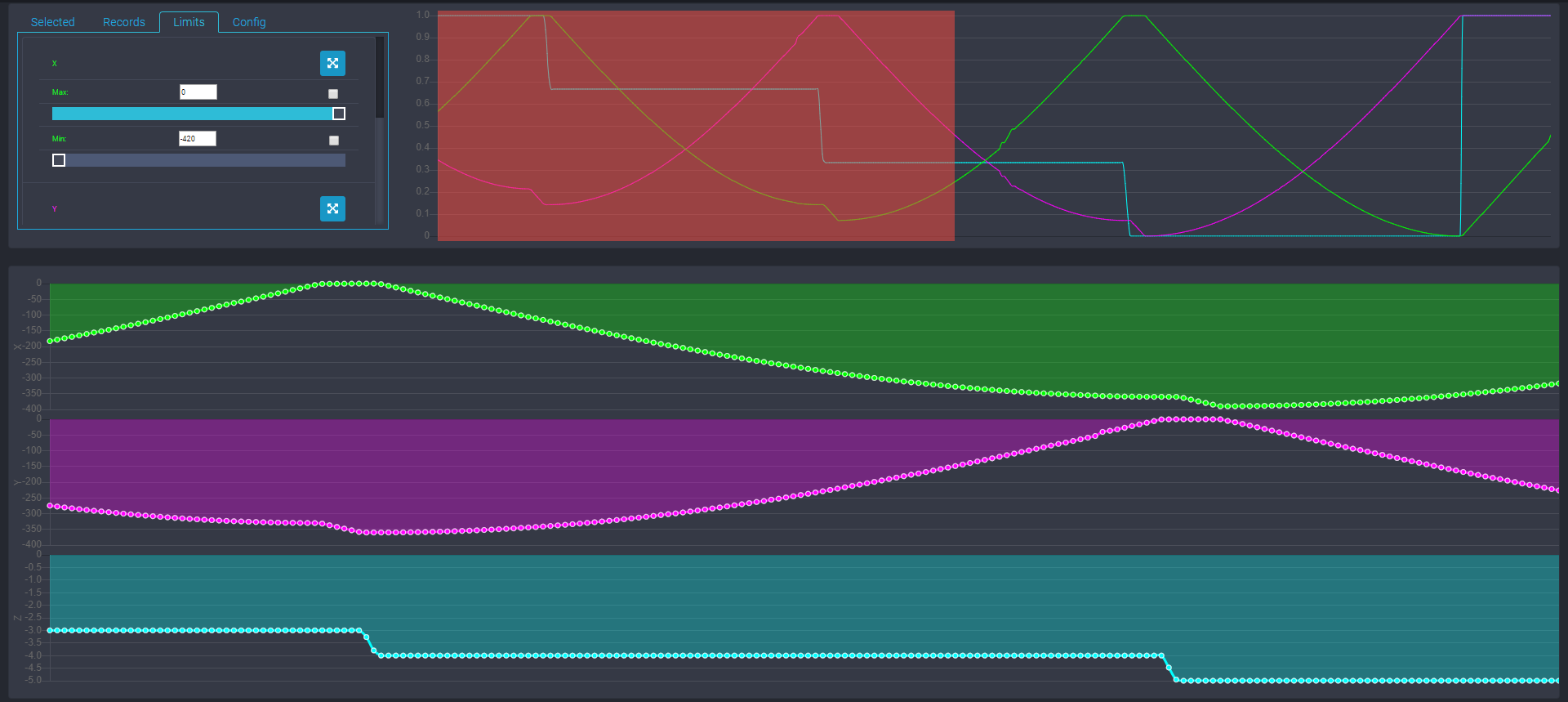
Development: weMonitor is used for test purposes during the development (completion of the sensor connection). In order to set up and adjust the machine optimally, the module is consulted to check the plausibility of the respective sensor values and whether they represent the process well enough. weMonitor clarifies among other things if the sensitivity is good enough, if the correct value range has been chosen, if the temporal and sensor value related resolution is high enough or if signal disturbances (sensor noise or process related disturbances) are to be detected.
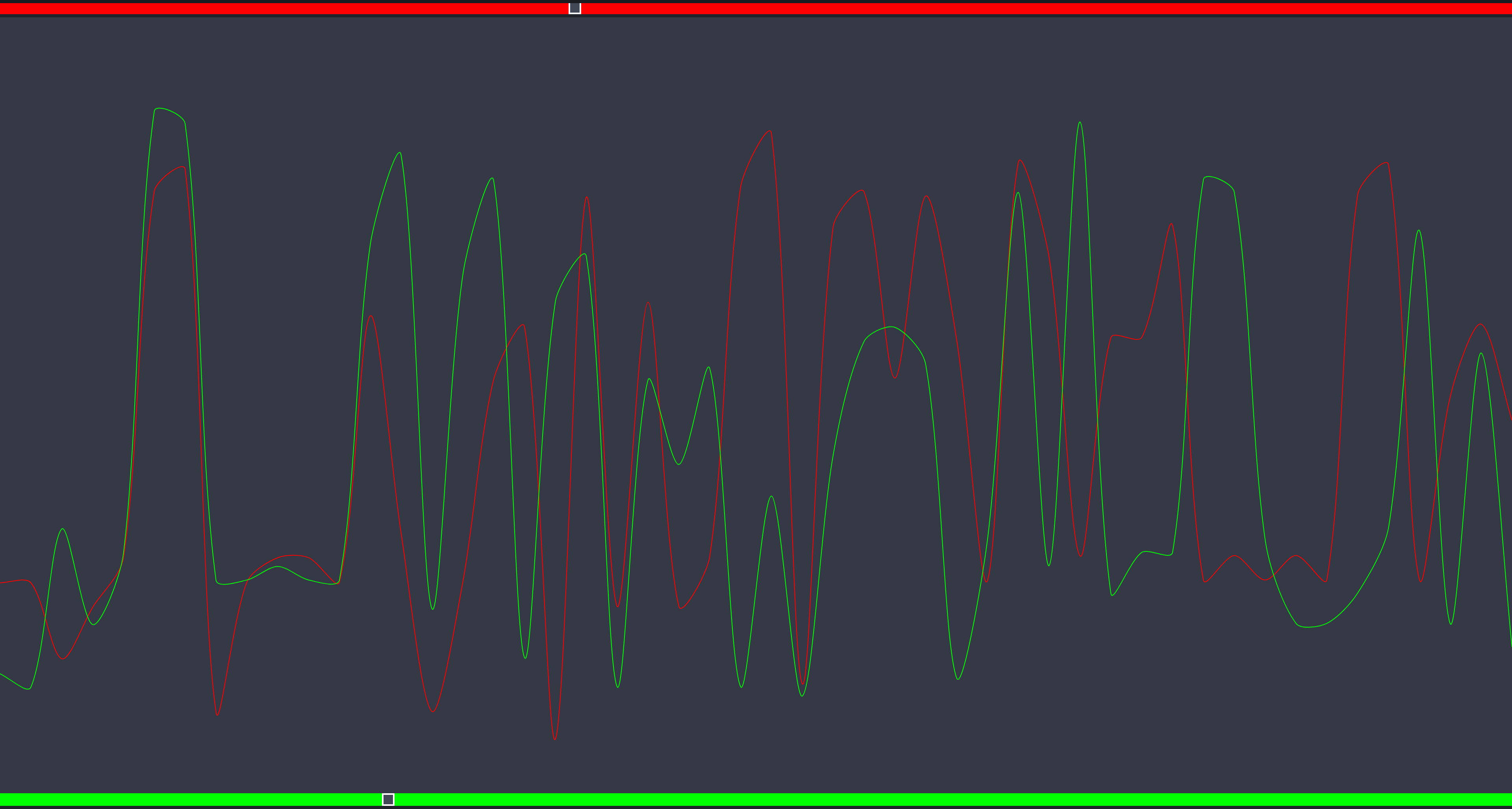
Initial Operation: weMonitor also supports the production of a machine series. In case of commissioning, the user falls back on a machine that is already optimally adjusted. This data is used to compare new machines with existing ones. Possible measuring deviations are thus detected quickly and can be corrected specifically. The comparison tool is also ideal for this purpose, as it compares the relevant measuring points and evaluates the deviations. In this way, the user can immediately see where corrections are required.
Running System: In the third scenario the signal analysis of weMonitor is used on a running machine or plant. By means of signal analysis a system is examined that is already operating in a given state. Based on the generated data the machine or plant can be examined directly. Thus developments, problems or errors can be detected even during operation. Subsequently, the users are able to take appropriate measures and improve the current operating status of the system.
The anomaly detection of weMonitor is a special version of the sensor monitoring and especially suitable for complex situations. In this application scenario a sensor pair is taught-in and an AI system is trained based on certain preset parameters. After completion of the learning phase this AI system monitors the corresponding sensors. However, the possibilities here go far beyond the comparison of target and actual values. The underlying AI and machine learning procedures describe and analyse machines and plants with complex threshold values as a coherent system. In this way, knowledge is generated independently, based on experience gained by the user. Predictive maintenance and servicing thus reaches a new level of performance, as the analysis of very large amounts of data is automated and appropriate solutions for new and previously unknown problems can be identified.
Example: The feed rate of a milling cutter is to be considered together with the power consumption of the same milling cutter. In a normal system, if the power consumption of the milling spindle remains below the preset limit, the machine seems to run optimally. In a complex system, however, the power consumption is measured in relation to the feed rate. This allows the system to determine whether the power consumption is also equal to the target in all speed ranges. A worn spindle bearing in the upper load range is difficult to detect from increased power consumption. However, if the feed speed is considered in relation to the power consumption, the system uses anomaly detection to detect this very well in the idle load range.
Further information on the underlying technology of anomaly detection can be found here.
Five arguments for weMonitor
Predictive maintenance of machines and plants ensures long-term reduction of maintenance costs
Predictive maintenance using machine learning prevents future machine failures and leads to significantly higher system availability
Extensive performance spectrum for continuous or discontinuous monitoring
Clear user interface with an informative display and analysis of alert messages
Support of high sensor sampling rates as well as real-time monitoring of machines and systems distributed worldwide
Back to module overview